
Aidison
Y2019
From 0 to 1, research to implementation
Aidison is a module in Datatist Marketing Cloud (DMC) web app, which applies AI-powered predictive models to campaign segmentation. It enable marketers to look into the future and focus on the right target at the right time. This case study is focusing on the MVP version.

lead product designer
I collaborated with the business manager, data scientists, and a development team to launch the MVP version. I led two designers to launch four more models after this project.
Jun.1.2019 - Aug.1.2019
Launched the MVP version
Problem
Through interviews and experiences of cooperations with clients, we found that most marketers are highly rely on analytics team to help them choose campaign segments, which affect the efficiency seriously when the analysts resource are limited.
Context
In the past 2 years, our data scientist team provided modeling service to optimizing campaign segments, which helped hundred companies increased conversion rate three to five times. In 2019, Datatist want to providing a trustable, scalable and automatic AI-powered segmentation solution to clients and to acquire more business partners.
Process
This project required deep understanding of domain knowledge of modeling and have very few influence product in the market. That's why I invest more time on rapid prototypes and getting feedbacks.
Phase 1 : Inspiration
-
Understand business goal and opportunities
-
Expert interview & secondary research
-
User interview & MVP scope
Phase 2 : Ideation
-
Brainstorm solutions
-
Prioritize user stories
-
Rapid prototype (Axure)
-
integrate feedbacks and iterate
Phase 3 : Implementation
-
Clickable low-fi prototype (Axure)
-
UI specs (sketch)
-
UX Documentation
Phase 1: Inspiration
Secondary research & expert interview
In order to communicate with clients professionally and be able to provide valuable solutions, I collaborated with data scientists to learn the process of all five models which we planed to implement in the next six months. To consider scalability, consistency, and reusability, I unified the different modeling process into 5 main steps.

Interviews & MVP Scope
I interviewed four marketers and one data analyst to understood their pain points of choosing and using segments in their current work process. I generated MVP scopes by using the JTBD method.

MVP Scope 1
Easily set up segment requirement
JTBD: When marketers try to set up a request for a segment via the product, marketers want to feel like they can easily understand the process, so they can spend less time on learning and remain productive.
MVP Scope 2
Choose segment with confidence
JTBD: Before marketers use the segment in their campaign process, marketers want to know why the segment is good, so they can trust the results and be confident in using the segment.
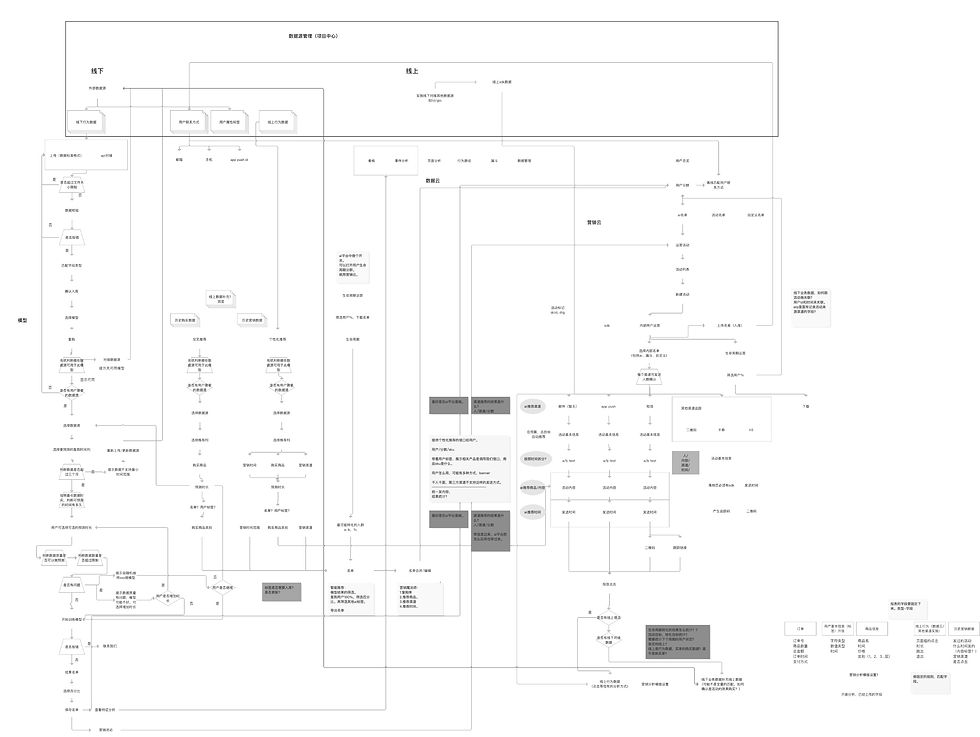
Phase 2: Ideation
Brainstorm Solutions & Prioritize User Stories
Aidison module as our core competence module, we are not only design it for Datatist Marketing Cloud (DMC) , but also want it to be able to integrated with other platforms easily, so that we can expand business with more partners. I collaborated with CTO and business manager work on the product and data structures, brainstorm potential solutions and narrow down to one model with limited user stories in MVP version.

Easily understand and used by marketers, as less steps as possible.
Abilities to adjust key model features

eg, when I choose to predict repurchase for certain products, I need to search by product name or code, so that I can quickly find it.
User Flow
From the interview, I found out there are just two main steps in marketers’ current job process. To match the users’ mental model, I regrouped and condensed the modeling process from 5 steps to 2 steps.

Prototype & iterate
Challenge 1: Transform technical setting to a story filling
For model set up, marketers need to determine what kind of user they are looking for regarding time and purchase behavior. I first sketched a wireframe and make sure the modeling process technically works, then matched the process to match marketer's mental model to make it understandable easily.
Before
model setting is focus on different time windows and target actions.

After
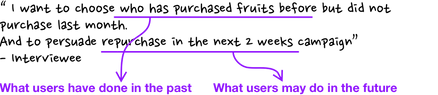
1. I reorganized three time windows into two to match marketers’ current job process
2.Pre-calculated the time window selection by weeks to match the database, so users will never fail
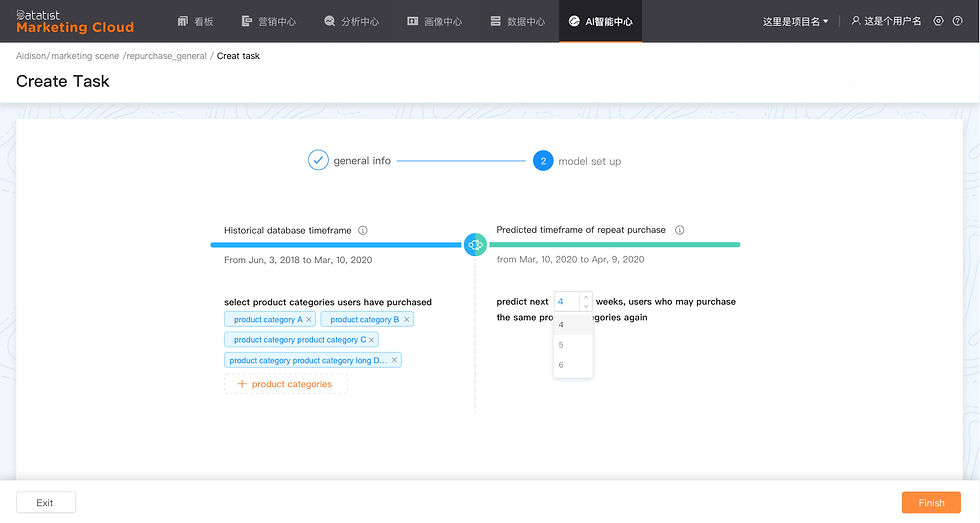
1
2
Challenge 2: Resolved conflicts with CDO by providing research data and a more functional solution.
After the model training is completed, marketers need to choose the segment based on model scoring to match their campaign strategy.
There are four sections related to verify and use the segment data regarding to original model process.
A: validate model performance by using historical data
B: Show user listing with predict model scores
C: Choose users with certain score range, and save as segment.
D: validate model performance by using updated data in the predict time window.
CDO's Concept : CDO consist the product should follow the original modeling process.
A
B
C
D
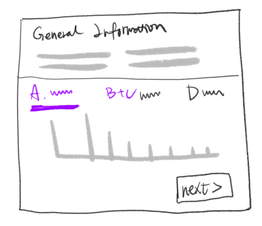
My Concept: I re-arranged and grouped the four section based on my assumption from user interview.

A
D
B
C
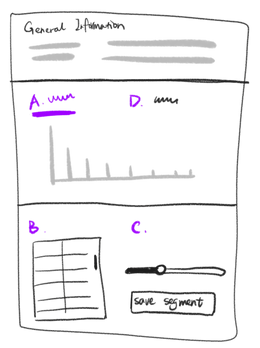
I created five small related tasks to test out the two solutions above with five marketers by using wireframe prototype. To my surprise, neither of the solutions work well. To find out why, I arranged a card sorting with testers.
Card Sorting
*different colors indicated different interviewees
I printed out each section per card and asked the 5 users to place them into the biaxial map and group them by their own view.
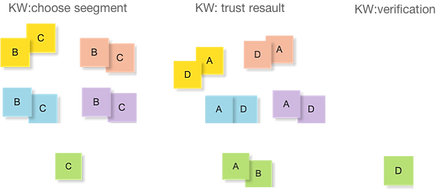
biaxial map
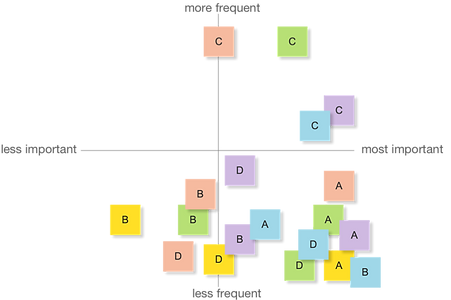
I provided user test result and card sorting insights with an iterated wireframe prototype to CDO, and proved the solution below is easier to understand and use.
C
B
A
D
1. The most important and frequent used function (C) was put to the top left, which is the first place eye drop on the screen.
2. Data in Section A would help to choose segment in section C, so A was placed under C, while users could still see it with C on one screen height.
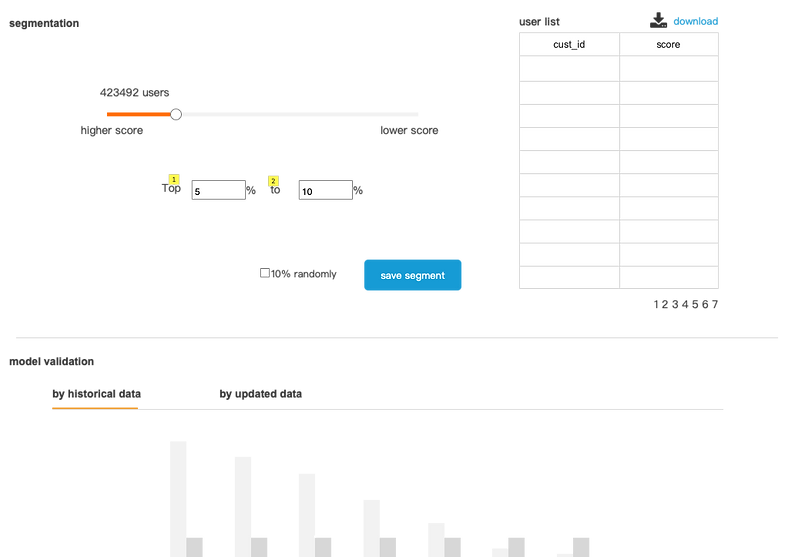
1
2
Challenge 3: Analyzed 20 slide widget, tested and iterated more than 10 times to make the experience of choosing a segment intuitively
Marketers need to choose the right users based on the ranking score to match their campaign strategy, so they have confidence to launch a campaign and improve the performance.
Before
Based on the previous interviews, business manager and I assumed that marketers would choose the top percentage of users with the highest score, which would maximize conversion rates. Users' scores were placed on the right hand as a reference.

However, after I test this function with marketers, I found their needs is differ from our assumption.
"I think the natural conversion rate is already good for the users with the highest scores, and we want to convert those with the middle scores." -Zoe (interviewee)
“I know that the higher the score, the more likely a user is to convert, but the "score" is abstract and hard to connect with my work” -Jone (interviewee)
After
1.convert the "scores" into practical meanings, such as purchase amount, which marketers use every day.
2. Marketers can choose any range of users with the corresponding data underneath.
3.Add the y-axis ordinate to help marketers clearly see the data range through
4.Collapse the user list. Because marketers can clearly see the score when using this slide, they don't have to look at the user list to make selection.
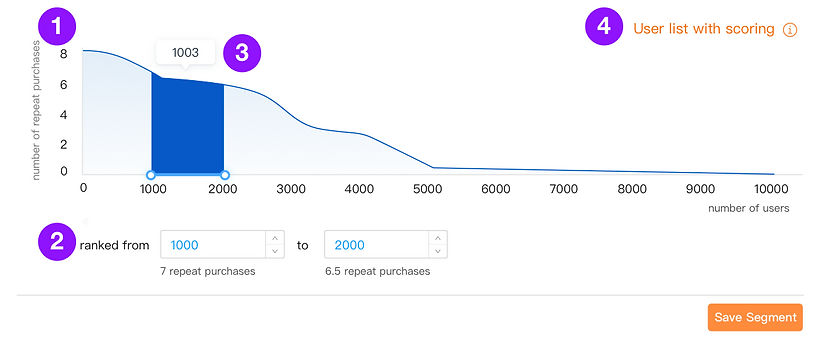
Phase 3: Implementation
The third phase is not just about "pixels perfect", but also to having regular check-in meetings with development team to make sure that the design fit into the technical constraints. As I already created a Design System, all the layouts, components, icons, typography, and colors are well defined, designers do not need to provide specs on those elements. Below are the selected examples of deliverables I hand to development team.
Selected Pages
design spec
I use sketch plug-in automatically generates accurate specs, assets, notes and code snippets from designs

IDD (Interaction Design Document)
I believe great design has to be complemented by equally good documentation.
Documentation for everyone involved in the development process is extremely important, it keeps team members receive the same information, help us collaborate more effectively. I documented scopes, product structures, user flows, interaction behavior, function, limitation and constraints.

Outcomes

Not only do users spend less time trying to understand how to create a segment, but they also spend less time performing this task, which improves their performance on their own KPIs.
For our clients
For Datatist Business

In one of China's biggest Insurance Groups, Aidison was used in one department and then expanded to 20 departments within half a year.
"Our active users exceeded 10 million per month for the first time. The Aidison module can be easily used by our marketers. Unlike other software we purchased which requires a lot of documentation and training. ”
-CMO of our client
Acquired 3 business partners
Include Alibaba Cloud, JD.COM, (top two online retailers in China) and Yonyou Software (one of the top software providers)